- HOME>
- EN>
- Research:
- Data Assimilation and Numerical Weather Prediction
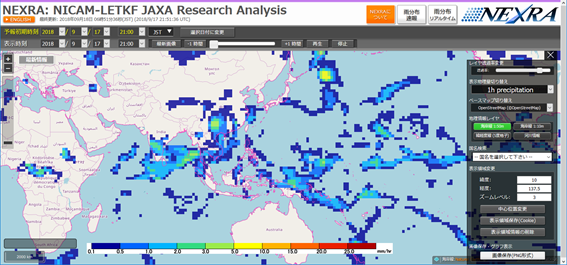
Significance of the Research
- Severe weather-related disasters, such as torrential rainfall, have become a global issue, increasing the importance of improving the accuracy of Earth environmental predictions, including weather forecasts. Over the past 50 years, the fields of meteorology and hydrology have advanced through the combined efforts of observational and theoretical research. Since 2000, with the development of computing technology, the era of numerical simulations has flourished, leading to development of numerous numerical models for weather and hydrological runoff analysis. As a result, computational techniques for precipitation, flooding, and inundation have significantly progressed. Subsequently, data assimilation has emerged as an important method for optimally integrating numerical models with real-world observations, playing a crucial role in advancing numerical weather prediction.
- Our laboratory is engaged in advanced research on weather forecasting using data assimilation. Data assimilation is not only used for state estimation and forecast improvement through the incorporation of observational data, but can also be applied to various purposes, such as evaluating the value of assimilated observations and estimating model parameters. In addition to numerical weather prediction models, we are also conducting research on data assimilation for land surface models and flood inundation models.
- In addition to simply applying data assimilation to numerical models, we are also engaged in mathematical research on data assimilation. For example, we specialize in advanced techniques such as the local particle filter and latent-space data assimilation, which are gaining attention as new data assimilation methods, as well as in accelerating data assimilation processes.
Selected Publications on Numerical Weather Prediction
- Kotsuki, S., Terasaki, K., Satoh, M., and Miyoshi, T. (2023): Ensemble-based Data Assimilation of GPM DPR Reflectivity: Cloud Microphysics Parameter Estimation with the Nonhydrostatic Icosahedral Atmospheric Model (NICAM), J. Geophys. Res., 128, e2022JD037447. doi: 1029/2022JD037447
- Kurosawa, K., Kotsuki, S., and Miyoshi, T. (2023): Comparative Study of Strongly and Weakly Coupled Data Assimilation with a Global Land-Atmosphere Coupled Model. Nonlin. Processes Geophys., 30, 457-479. doi: 10.5194/npg-30-457-2023
- Kotsuki, S., Sato, Y., and Miyoshi, T. (2020): Data Assimilation for Climate Research: Model Parameter Estimation of Large Scale Condensation Scheme. J. Geophys. Res., 125, e2019JD031304.
Selected Publications on Data Assimilation (methodological)
- Oishi, K., and Kotsuki, S. (2023): Applying the Sinkhorn Algorithm for Resamling of Local Particle Filter, SOLA, 19, 185-193. doi: 10.2151/sola.2023-024
- Kotsuki, S., Miyoshi, T., Kondo K., and Potthast R. (2022): A Local Particle Filter and Its Gaussian Mixture Extension Implemented with Minor Modifications to the LETKF. Geosci. Model Dev., 15, 8325–8348. doi: 10.5194/gmd-15-8325-2022
- Kotsuki, S., and Bishop, H. C. (2022): Implementing Hybrid Background Error Covariance into the LETKF with Attenuation-based Localization: Experiments with a Simplified AGCM. Mon. Wea. Rev., 150, 283-302. doi: 1175/MWR-D-21-0174.1