- HOME>
- EN>
- Research:
- Disaster Prediction with AI and Data Science
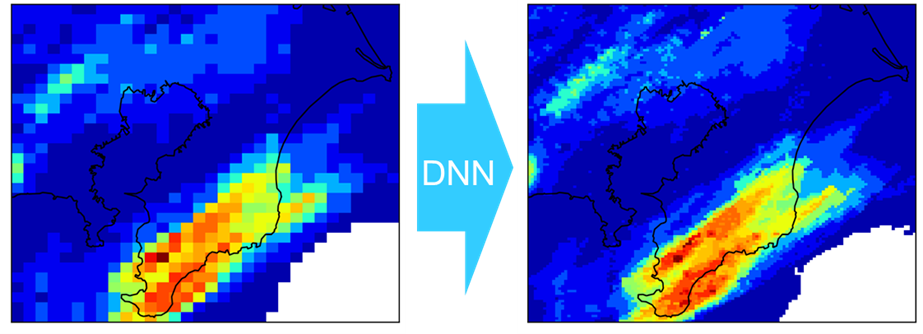
Significance of the Research
- Empirical sciences have evolved through three paradigms: observational science → theoretical science → computational science, and we are now in the era of the fourth paradigm—data science (Jim Gray, 2007). Japan's flagship supercomputer, Fugaku, which became operational in 2020, boasts 100 times the computing power of its predecessor, K computer. In addition to large-scale parallel computing for meteorological simulations, it is also designed to facilitate machine learning and AI development. Japan is actively building a research environment that integrates high-performance computing with data science.
- In Japan, severe water-related disasters—such as typhoons and linear rainbands—are becoming increasingly problematic, likely due to climate change. Torrential rains, floods, and inundations occur almost annually, with 2019 recording the highest-ever flood damage cost of 2.15 trillion yen. Enhancing the accuracy of meteorological and hydrological predictions for extreme rainfall and floods—and utilizing this information for control and mitigation strategies, such as dam operations—has become a critical societal mission.
- Our laboratory is advancing disaster prediction research using cutting-edge deep learning techniques. We focus on predicting extreme precipitation, accelerating flood inundation simulations, and enabling early detection of wildfires. Additionally, we are developing technologies to maximize the value of observational data, including data compression and phenomenon interpretation for geoscientific datasets through data science.
Selected Publications on AI Weather Prediction
- Kotsuki, S., Shiraishi, K. and Okazaki, A. (2024): Integrating Ensemble Kalman Filter with AI-based Weather Prediction Model ClimaX.
Selected Publications on AI-based Disaster Predictions
- Momoi, M., Kotsuki, S., Kikuchi, R., Watanabe, S., Yamada, M., and Abe, S. (2023): Emulating rainfall-runoff-inundation model using deep neural network with dimensionality reduction. Artificial Intelligence for the Earth Systems (AIES), 2, 1-25. doi: 10.1175/AIES-D-22-0036.1
- Hu, J., Kotsuki, S., Igarashi, Y., Yang, Z., Talerko, M., Tischenkof, O., Protsak, V. and Kirieiev, S. (2024): A tuning-free moderate scale burned area detection algorithm — A case study in Chornobyl contaminated region. Int. J. Remote Sens., 45, 2444-2461. doi: 10.1080/01431161.2024.2331976
- Hu, J., Igarashi, Y., Kotsuki, S., Yang, Z., Talerko, M., Landin, V., Tischenko, O., Zheleznyak, M., Protsak, V., and Kirieiev, S. (2023): Application of a tuning-free burned area detection algorithm to the Chornobyl wildfires in 2022. Sci. Rep., 13, 5236. doi: 10.1038/s41598-023-32300-5
- Ouyang, M., Kotsuki, S., Ito, Y., and Tokunaga, T. (2022): Employment of hydraulic model and social media data for flood hazard assessment in an urban city. J. Hydrol. Reg. Stud., 44, 101261. doi: 10.1016/j.ejrh.2022.101261
Selected Publications on Data Science
- Oettli, P. and Kotsuki, S. (2024): An Objective Detection of Separation Scenario in Tropical Cyclone Trajectories Based on Ensemble Weather Forecast Data. J. Geophys. Res., 129, e2024JD040830. doi: 10.1029/2024JD040830