- HOME>
- EN>
- Research:
- Global Precipitation Mapping and Global Precipitation Measurement Mission
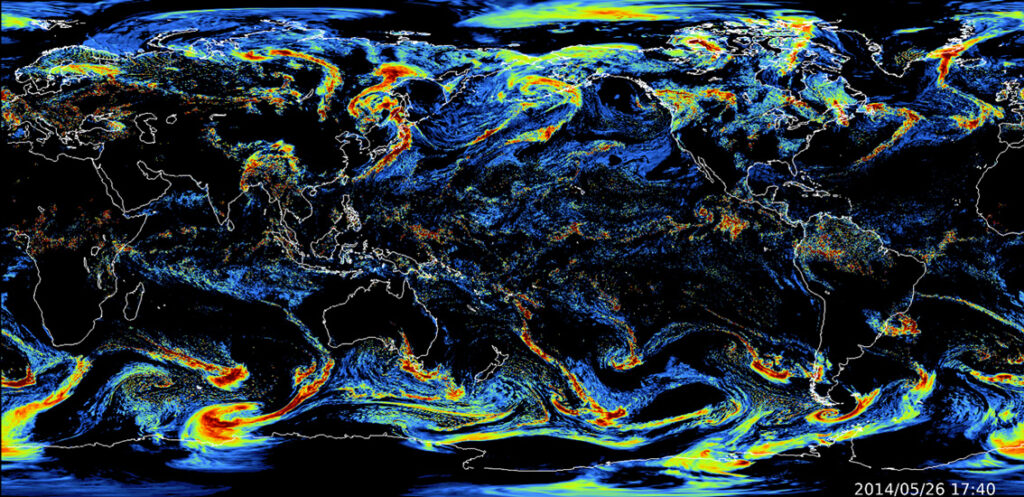
Importance of the Research
- In recent years, severe water-related disasters such as typhoons and linear rainbands, which are believed to be influenced by climate change, have become a major global issue. Japan also experiences heavy rainfall, floods, and inundations almost every year, making it an urgent priority to improve the accuracy of meteorological and hydrological monitoring for extreme rainfall and floods. To address the global challenge of water-related disasters, estimating global precipitation using satellite observations covering the entire Earth is not only essential for the advancement of Earth sciences but also holds significant societal importance.
- Our laboratory is working on the enhancement of JAXA's Global Satellite Mapping of Precipitation (GSMaP) by integrating Global Precipitation Measurement (GPM) and other satellite observation data with land surface data assimilation and machine learning techniques. The GSMaP algorithm is based on microwave radiometer and microwave sounder precipitation estimates (GSMaP_MWR), which are trained using the Precipitation Radar (PR) onboard the Tropical Rainfall Measuring Mission (TRMM) satellite and the Dual-frequency Precipitation Radar (DPR) onboard the GPM core satellite. Additionally, advanced GSMaP products have been developed, such as GSMaP Moving Vector with Kalman Filter (GSMaP_MVK), which applies spatiotemporal extrapolation of non-observed areas using rain area movement vectors (Ushio et al., 2009), its near real-time version GSMaP Near Real Time (GSMaP_NRT), and GSMaP_Gauge, which applies daily rainfall correction using ground-based precipitation observations from the NOAA Climate Prediction Center (CPC) (Mega et al., 2018).
- Further improving the accuracy of the highly developed GSMaP requires leveraging data science techniques that have not been fully utilized in existing research. Our research aims to enhance the GSMaP algorithm by integrating land surface models, data assimilation, and machine learning, spanning physical sciences, statistical mathematics, and information science. With the anticipated increase in Earth observation satellites, developing advanced data integration technologies will become even more critical. Our laboratory is pioneering a data-driven, satellite-based global precipitation mapping system that does not rely on computationally expensive numerical models by making full use of cutting-edge AI technologies.
Selected Publications
- Muto, Y. and Kotsuki, S.(2024): Estimating global precipitation fields by interpolating rain gauge observations using the local ensemble transform Kalman filter and reanalysis precipitation. Hydrol. Earth Syst. Sci., 28, 5401–5417. doi: 10.5194/hess-28-5401-2024 (Dec. 17, 2024) [Link to Press Release in English]
- Muto, Y., Kanemaru, K., and Kotsuki, S. (2023): Correcting GSMaP through histogram matching against satellite-borne radar-based precipitation, SOLA, 19, 217-224. doi: 10.2151/sola.2023-028